
Implementing sensor-based digital health technologies in clinical trials: Key considerations from the eCOA Consortium
Originally appeared in Clinical Translational Science, November 2024
By: Elena S. Izmailova, Danielle Middleton, Reem Yunis, Julia Lakeland, Kristen Sowalsky, Julia Kling, Alison Ritchie, Christine C. Guo, Bill Byrom, Scottie Kern
Abstract
The increased use of sensor-based digital health technologies (DHTs) in clinical trials brought to light concerns about implementation practices that might introduce burden on trial participants, resulting in suboptimal compliance and become an additional complicating factor in clinical trial conduct. These concerns may contribute to the lower-than-anticipated uptake of DHT deployment and data use for regulatory decision-making, despite well-articulated benefits. The Electronic Clinical Outcome Assessment (eCOA) Consortium gathered collective experience on deploying sensor-based DHTs and supplemented this with relevant literature focusing on mechanisms that may enhance participant compliance. The process for DHT implementation starts with identifying a clinical concept of interest followed by a digital measure selection, defining active or passive data capture and their sources, the number of sensors with respective body location, plus the duration and frequency of use in the context of perceived participant burden. Roundtable discussions among patient groups, physicians, and technology providers prior to protocol development can be very impactful for optimizing trial design. While diversity and inclusion are essential for any clinical trial, patient populations should be considered carefully in the context of trial-specific aims, requirements, and anticipated patient burden. Minimizing site burden includes assessment of training, research engagement, and logistical burden which needs to be triaged differently for early and late-stage clinical trials. Additional considerations include sharing trial results with study participants and leveraging publicly available data for compliance modeling. To the best of our knowledge, this report provides holistic considerations for sensor-based DHT implementation that may optimize participant compliance.
INTRODUCTION
In this manuscript, we refer to the US Food and Drug Administration’s (FDA) definition of a digital health technology (DHT)1 and specifically focus on sensor-based DHTs that constitute sensor-based hardware and firmware coupled with data processing algorithms to collect health-related information in an objective way.
The early phase of adoption of sensor-based DHTs for use in clinical research gained interest rapidly and was characterized by conceptual thinking, a launch of pilot experiments,2 and hype.3 Despite limitations, the learnings from earlier efforts proved valuable and enabled the development of frameworks4, 5 to design and execute DHT-enabled experiments. This, in turn, facilitated the deployment of sensor-based DHTs in natural history studies6, 7 aimed at creating digitally enabled reference datasets to inform clinical proofs of concept, as well as in interventional studies to attain practical experience of using these tools in consort with other clinical trial procedures and inform digital end-point use in future trials.8-10
Some sensor-based DHTs have gained regulatory endorsement11-13 and some incorporated into ongoing14 and completed trials, including the FDA agreement for using an actigraphy-derived measure to support a primary end point in a Phase III trial,15 but the total number of studies deploying these technologies has been relatively small and indicates a slower rate of DHT adoption. The limited deployment of DHTs in clinical research is surprising given the potential of DHTs as described earlier16-18 and alongside the objectively assessed financial benefits.19 Moreover, adoption of remote data collection as a part of decentralized clinical trials was accelerated during the COVID-19 pandemic with the support of appropriate regulatory guidance.1, 20 Consequently, one could reasonably anticipate an increase in the number of clinical trials using sensor-based DHTs with the hope of solving many of the challenges that plague clinical trial execution, including long durations and high cost due to the need to recruit relatively large number of clinical sites and participants21 to power a trial appropriately, participant retention and their ability to complete clinical trial procedures that would contribute to a final dataset.22
Several reasons have been identified as contributors to the lack of a wider adoption of DHTs in clinical research. Of principal concern are those outstanding regulatory questions pertaining to evidentiary package expectations for sensor-based DHT-derived measures,23 a concern that could be addressed by generating evidence to enable further development of regulatory guidance and precompetitive work to align DHT-related terminology.24 In addition, clinical trial sponsors exhibit a hesitation attributable to a fear of potential deployment challenges with these relatively new technologies which, in turn, could conceptually hamper evidence-generating studies and further development of regulatory science. Sponsors’ concerns often pertain to the site’s and/or participant’s ability to manage such technologies and the risk of an associated burden that might impact on the generation of valid data, and, ultimately, qualified measures. Questions like—“How do we know that participants will use these devices correctly at home? Would adding DHT-derived measures increase the overall burden on a participant? What is the anticipated compliance? Are there any data on participant compliance with a particular device in a given disease population? Can study participants manage the technologies including battery charging, data synchronization, using devices properly?”—are asked in preparation for almost any trial for which a decision has been made to explore the use of DHTs. Successful deployment of DHTs is often measured by participant compliance in wearing a sensor and generating valid data.
We define compliance, which sometimes is also termed adherence,25 according to the National Cancer Institute Definition of Terms as “the act of following a medical regimen or schedule correctly and consistently, including taking medicines or following a diet”.26 A recent systematic review of sensor-based DHT-derived participant compliance indicated tremendous variability in both defining and reporting compliance.25 There are no established and commonly accepted thresholds of acceptable compliance, though some authors perceived 75%–80% compliance as good or satisfactory whereas rates above 90% were perceived as high or very high.27, 28 However, compliance is very context dependent and can be impacted by a number of factors.
To answer those questions frequently asked during the trial design phase, the members of the Critical Path Institute’s Electronic Clinical Outcome Assessment (eCOA) Consortium identified key topics based on these frequently asked questions, summarized authors’ collective experience with sensor-based DHT deployment and supplemented it with findings and conceptual thinking gleaned from peer-reviewed literature and relevant regulatory guidance documents, resulting in the derivation of the considerations summarized in Figure 1. Furthermore, these considerations reflect on approaches that optimize participants’ compliance with using DHTs per protocol. Although not all the below-described steps directly impact upon study participants’ compliance, it is important to outline the study preparation steps to develop a complete picture (Figure 1).
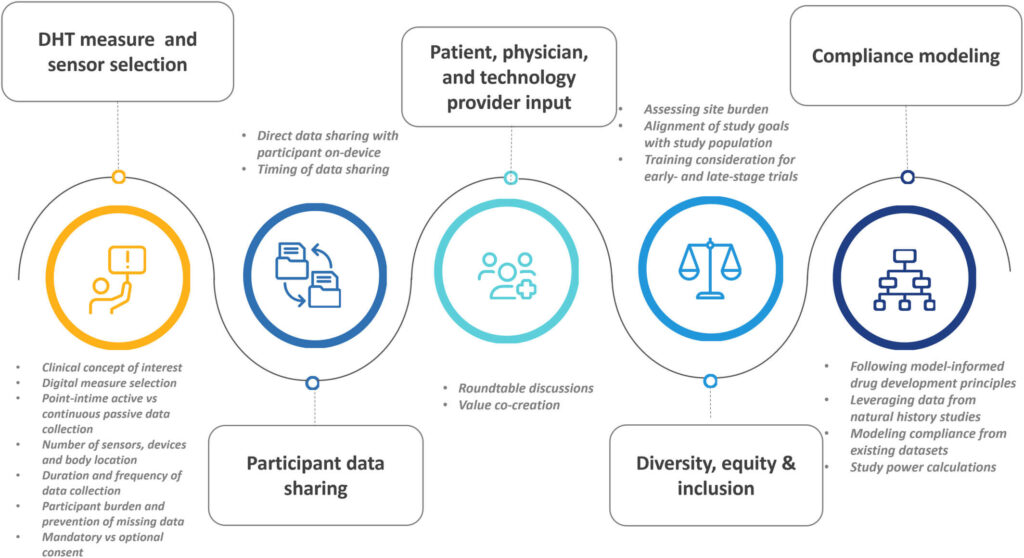
DEFINING A CLINICAL CONCEPT OF INTEREST AS A STARTING POINT
The selection of DHTs and related measures starts with identifying a clinical concept of interest that is relevant to the participant, rather than identifying the technology itself; this starting point empowers compliance by defining a characteristic that the trial participant will experience and be able to report. The selection of digitally derived measures and related DHT-based candidates, including hardware, firmware and software (Figure 1) is the second step. However, it is critical to note that identifying clinically meaningful concepts is linked to patients’ belief that the measure effectively evaluated an important symptom; this dynamic was ably demonstrated in early Parkinson’s disease, with a described “greater participant engagement and reciprocity.”29
DHT-derived measures can be classified as biomarkers or eCOAs; the exact classification is dependent on the context of use.23, 30 In the case of biomarkers, candidate measure development starts with describing the unmet medical need, why a biomarker is needed and what role it will play in addition to the existing tools and assessments in drug development efforts.31 Examples of unmet medical need include cardiac monitoring for extended periods of time outside of clinical pharmacology units,32 the need to detect frequent changes in a disease condition as a result of diurnal variation and environmental exposure,33 and biomarkers to identify cytokine release arising during certain anticancer treatments.34 Statistical considerations for DHT-based monitoring for adverse events are discussed elsewhere.32 In the case of eCOA, the measure development begins with identifying aspects of health that are meaningful to patients. The supporting methodology has been thoroughly outlined in recent FDA guidance on patient-focused drug development.35 Examples of DHT-based eCOAs are: mobility as measured by an appropriate body-worn DHT in Duchenne muscular dystrophy (DMD),11 or assessment of motor function in Parkinson’s disease (PD) via more sensitive digital measures of movement not granularly captured via traditional clinical scales.7, 8, 36 In some cases, both biomarkers and eCOA may be needed to obtain a full picture of a patient’s condition. For instance, asthma status can be captured by both biomarkers obtained by means of mobile spirometry33 and electronic patient-reported outcome (ePRO) measures capturing disease symptoms during the day and night.37 Other regulatory considerations can be found in the review by Bakker et al.38
Digital measure selection
Once a biomarker or an eCOA-based concept of interest is selected, the next step includes determining whether it can be captured by means of sensor-based DHTs (Figure 1).
Some disease symptoms are more amenable to characterization by digital means than others. For example, an impairment of gait, balance, and motor function can be quantified using body-worn inertial sensors such as accelerometers, gyroscopes, and magnetometers.39-42 In other diseases, key symptoms can be difficult to capture by sensor-based methods. For example, the best practices for capturing gastrointestinal symptoms rely on patient reports.43 Considerations for selecting a particular sensor-based DHT for a specific purpose are described elsewhere44, 45 and include the DHT’s form factor, body placement, device battery, data synchronization process, data processing algorithms, and the associated burden on the participant. Below, we provide additional considerations not described in detail in the peer-reviewed literature (Figure 1).
Point-in-time active or continuous passive data capture
Sensor-based DHT-derived measures can be derived, point-in-time, predefined tasks which require active participation or employ passive continuous monitoring. Examples of such measures are shown in Table 1. Some point-in-time measures are considered performance outcome (PerfO) measures and may increasingly be implemented using sensors, both in-clinic and at home. Examples are included in the recent ISPOR good practices task force report on recommendations on the selection, development, and modification of PerfO assessments.46 The determination of point-in-time active versus continuously collected passive measures is often driven by the concept of interest defined in the protocol. For example, if the clinical concept of interest is overall daily activity, passive measurement capturing physical activity across the majority of the waking day may be pertinent. On the other hand, if specific gait parameters are more relevant, these may be more suited to point-in-time PerfO measures where patients, for example, conduct a short walking test for long enough to reliably assess the gait parameter of interest. In general, however, passive measures capture what a patient elects to do, whereas PerfO measures capture what they are capable of doing. The two are similar, but not the same, as indicated by a recent systematic review of the IDEA-FAST initiative for DHT-enabled fatigue measures.47
TABLE 1. Examples of active point-in-time and continuous monitoring measures.
Description and reference | Type of measure (point in time or continuous) | Biomarker or COA | Reported compliance |
---|---|---|---|
Mobile spirometry performed daily or twice a day in asthma (Huang et al., 2020)33 | Point in time | Biomarker | 69.9% twice-daily measurements within prespecified windows, 85.3% once daily |
Ambulatory heart rate monitoring in healthy volunteers (Izmailova et al., 2019)55 | Continuous | Biomarker | 53%–95% during the stay in the clinical pharmacology unit; 69%–96% at home |
Moderate to vigorous physical activity in fibrotic interstitial lung disease (King et al., 2022)10 | Continuous | eCOA | Not reported |
Detection of PD motor signs by means of a smart phone (Lipsmeier et al., 2022)104 | Point in time | eCOA | On average, daily remote active testing took a median of 5.3 min on days without the Symbol Digit Modalities Test (SMDT), and 7.32 min on days with SDMT. Average adherence was high with 96.29% (median per participant) of all possible active tests performed during the first 4 weeks of the study (i.e., 26–27/27 days). Participants contributed a median of 8.6 h/day of study smartphone and a median of 12.79 h/day of study smartwatch passive monitoring data |
The deployment of both point-in-time active tasks and continuous remote data collection approaches requires careful consideration (Table 2). The variability of data collected in an unsupervised environment presents greater challenges compared to data collected at supervised clinic visits where greater standardization can be imposed, and particularly relevant when participants are required to follow specific data collection protocols.48 Study participants can experience challenges with managing technologies on their own which may result in variable participant data contribution.25 Moreover, both point-in-time and continuous measures may require contextual data to support interpretation of results—this can be achieved with patient-completed questionnaires with predefined content,49, 50 or potentially by means of diaries of semi-structured or even unstructured format,51 though unstructured data collection from participants is not currently considered a standard practice. Additionally, use of advanced data analytics may be required to fully leverage the large amounts of data.32, 52
TABLE 2. Considerations for DHT-based measures collected in the clinic and remotely (point-in-time active tasks and continuous passive data monitoring.
Setting | Pros | Cons |
---|---|---|
In the clinic |
|
|
Active tasks performed remotely |
|
|
Remote passive monitoring |
|
|
Ultimately, the amount of data required to answer questions posed by a study needs to be considered in the context of DHT choice and specific measure selection. Often, passive data collection is considered to be of lower burden for patients as compared to active point-in-time tasks. However, in some instances, point-in-time active measures can be less burdensome for participants than continuous monitoring. For example, in a study designed to compare continuous versus intermittent measurement via electrocardiogram for atrial fibrillation screening in elderly people, compliance for continuous measurement by a wearable device worn around the neck was lower than that of the intermittent measurement four times daily via a hand-held device.53
Number of sensors, technologies/devices, and body location
The ability to collect data from multiple sensors, either multiple sensor modalities on the same technologies or multiple distinct sensors attached to different body locations, could enhance the accuracy and robustness of the targeted digital measure.54 The deployment of multiple sensors, however, might increase site or participant burden, either by the need to wear those sensors on multiple body locations, or the need to charge devices frequently due to higher power consumption. This tradeoff between data requirements and burden needs to be carefully balanced, especially for large-scale, multisite trials which face very different operational challenges than small or single-site studies.
During the early days of the COVID-19 pandemic, the use of sensor-based DHTs for remote data collection sharply increased, necessitating a reassessment of devices and data quality, consideration of limitations of different form factors and body placements, as well as the number of devices and measurements. Vital sign monitoring sensors are relatively well established and understood by both the scientific community and general public.44 However, for some measures, deployment of multiple sensors is required; for example, concurrent measurement of accelerometry data enables better interpretation of ambulatory heart rate data which is labile and may change rapidly depending on the level of physical activity.55 Sensor systems that automate the time synchronization of data from each sensor are important to avoid the complications associated with time alignment when looking to combine the data collected using multiple, independent sensors.
A novel, evolving field of DHT applications in clinical research involves an assessment of multiple concepts of interest by means of accelerometers and gyroscopes which can be embedded in actigraphy devices or smartphone-based inertial measurement units (IMUs). Gait and balance, which are impaired in both neurological56-58 and musculoskeletal conditions,11 can also be assessed using body-worn sensors, and there are multiple options available in terms of the number of sensors deployed,59 body placement, and form factors60 either for passive monitoring in free living settings or during performance of active tasks in the clinic or in a controlled remote setting.59 Deployment of multiple body-worn sensors to assess gait and balance in participants captures detailed data that can provide the high levels of sensitivity in specific disease indications,61, 62 although this configuration may be better suited for assessments in the clinic or a controlled remote setting where data are collected while participants are performing defined tasks under supervision (live or virtual).59 A comparison of data derived from six devices and a single lumbar mounted device demonstrated excellent and good agreements in PD and healthy participants for a subset of features of gait and balance during a 2-min walking task.63
Standards apply to the optimal location of the device on the patient’s body and may be driven by the balance of wear compliance/convenience and measurement science in relation to the specific outcome measure of interest.64 For example, common sensor locations for accelerometers measuring physical activity/gait include the wrist, arm, waist, thigh, and foot. The wrist may be chosen for patient convenience and maximizing wear time, whereas the ankle may be more suited to accurate estimation of stepping, the thigh for more robust information on sit/stand transitions (and therefore sedentary behavior), and the waist for optimal estimation of energy expenditure associated with walking activity.65
While several groups have reported an optimal body placement of the IMUs at the waist,66 patient preference, and acceptability are important. For example, a study of adolescent children wearing an accelerometer showed that wear compliance was significantly higher with wrist placement compared to hip placement, with comfort and embarrassment being cited as major reasons for favoring the wrist.67 Complex data collection, consisting of point-in-time and continuous data, should be considered carefully for long-duration assessments, for example, efficacy data collection in slowly progressing diseases, assessment of ease of use/data sensitivity trade-offs, also requiring formative human factor and usability studies to inform a final solution for deployment.
Duration and frequency of data collection
The duration of DHT use is one of the factors impacting participant compliance. A decrease in DHT wear time and subsequent data generation over time has been documented.27, 68 A decrease in compliance from 94% initially to 85% over 30 days was reported in older adults wearing a fitness tracker for step tracking post cardiac surgery.69 While the overall compliance for continuous use of a fitness tracker in another study testing the deployment in breast cancer participants was 52.5% over 6-month follow-up, the longitudinal compliance rate rapidly decreased over time, reaching 17.5% at day 180.70
The duration of data collection is driven by the trial end point, which dictates a minimum time period, for example, 1 year is necessary to detect progression in PD.71 It is also related to the concept of a minimum valid data set, which is defined as the amount of data required to enable the estimation of the health outcome(s) of interest to the degree of accuracy deemed appropriate and avoid collecting unnecessary data which may increase participant burden.72 The Mobilise-D project, run under the auspices of European Innovative Medicines Initiative defined a minimal data set required to determine reliable digital measures of walking activity and gait by collecting data from participants with medical conditions, characterized by walking impairment and higher than average risk for falls which include chronic obstructive pulmonary disease (n = 559), multiple sclerosis (n = 554), Parkinson’s disease (n = 542), and proximal femoral fracture (n = 469). Study participants were asked to wear a DHT for 24 hours a day for a continuous period of 7 days. First, the minimum number of hours a device needed to be worn during a single day to determine if a particular day can be considered valid was established. This minimal daily wear time ranged between no minimal requirement and >14 hours, depending on the measure. Second, the number of required valid days was determined for each walking activity and gait parameter to obtain a reliable value. This minimal daily wear time number ranged between 1 day and >1 week. If all parameters for all conditions under consideration are examined within the same study, the proposed minimal daily wearing time of >14 hours during waking hours to obtain valid days, and at least 3 valid days to obtain reliable parameter values.73
If a DHT-derived measure constitutes a safety consideration, such as mobility impairment from chemotherapy-induced peripheral neuropathy in oncology trials,74 sufficient time should be allocated to collect data to detect such a signal. In addition to disease-specific considerations, duration and frequency of data collection can be dictated by the choice of a combination of a sensor and a data processing algorithm. For example, a minimum duration of sleep data collection by means of an actigraphy device has been described and details both a minimum baseline period and a specified timeframe for assessment after commencement of treatment in the context of interventional studies.75 Additionally, the frequency and the duration of data collection should be considered according to both a measure’s variability over time and the DHT’s technical performance (verification and validation1). This can be addressed by statistical power calculations which involve modeling that takes into account measure variability and the number of measurements.33 In practice, given the nascence of this space, such information might not be available. Study teams might need to make certain assumptions based on the best available information in the literature and collect enough data to better understand the measurement properties and make data-driven decisions.
The other key consideration is to accurately capture the concept of interest. For example, the overall physical activity, which can be a step count or a number of physical activity bouts per day,76 may need to be recorded for most of the hours when patients can walk and preferably capture both weekdays and weekends, if activity patterns are likely to differ. On the other hand, collecting an aspect of gait, like walking speed or stride velocity, requires a small number of bouts of sustained activity to be measured for each day to be valid. These approaches also require defining a valid day and the number of valid days to assess the adequacy of an estimation of the outcome measure.
Participant burden and prevention of missing data from DHTs
DHT deployment in clinical trials is often perceived as an additional burden to participants and a complicating aspect of trial conduct. However, it is important to separate disease-related burden from the burden of DHT use. In many chronic health conditions, the two concepts may be conflated, as technology use may present an additional burden to an already challenged participant population. In contrast, the ease of wearable technology use may alleviate disease burden by enabling certain medical visits to be conducted remotely with supplemental physical activity or physiological data36 providing novel insights into day-to-day disease changes.77 A large component of burden is the patient’s perception of their health condition counterbalanced by the utility of a proposed technology and its accuracy.78 Whitelaw et al. conducted a systematic scoping review of barriers and facilitators to the uptake of DHTs which identified that easy to use technology, previous experience, perceived usefulness, and empowerment as key factors that enable the adoption of DHTs, meanwhile difficulties with technology use, technical problems, certain medical conditions, such as mental health, and the lack of interest are barriers that might contribute to a perceived participant burden.79
The concept of participant burden is closely related to patient data contribution and data missingness.50 Limiting the quantity of missing data is an important consideration during study planning. Despite the use of statistical approaches to handle missing data,50 too much missing data mean that inferences based on the sensor/wearable data may be considered insufficiently robust to be reliable. Reducing the likelihood of missing data is therefore an important consideration. Researchers can consider three components to limiting missing data:
Ensuring acceptable usability and feasibility of the sensor-based DHT80 prior to study initiation
Researchers should select DHTs that have good usability properties with the specific patient population in mind. In some cases, this may require usability testing beyond that made available by the device manufacturer, especially if the study context of use is different from the intended use on the device label. Furthermore, feasibility research may be required to understand whether the DHT use in the context of the protocol is likely to present problems for participants—for example, whether the frequency/duration of use is considered sufficiently burdensome to affect compliance.
Providing thorough training to all participants (see also site burden and training considerations)
Participants should be trained to use the DHT, so that they are able to use it as required by the study, for example at home, without supervision. It is strongly recommended to explain the importance of the data to each participant to motivate compliance.
Proactive monitoring of sensor-based DHT data compliance during the study
The choice of a particular sensor and data processing software which may include a software development kit (SDK) may be amenable to data streaming directly into a service provider’s cloud environment that in turn enables visualization and monitoring of compliance in near real time by the study site. In this case, automated alerts and reminders can be triggered to participants and sites when compliance falls below a defined threshold. Furthermore, notifications and reports may facilitate sites’ proactive data monitoring and participant remediation.
Mandatory versus optional consent
As discussed above, the design of DHTs in the study protocol requires a careful balancing between the amount and quality of data and participant’s burden, with the goal to optimize participant compliance. Because sensor-based DHT data are often used to support exploratory objectives in interventional studies, study teams might be tempted to make their use optional to reduce burden. Unfortunately, this approach most often leads to lower participant willingness to participate, perceived lower data value, and subsequently lower compliance with DHT use and consequently insufficient data to answer research questions. It is important to note that such consequences are not unique to DHTs. Any study components made optional could be perceived as not important by participants and sites. An alternative approach includes designing a specific substudy with mandatory DHT use. As studies that ended up with very low compliance for data collection are often not reported in the peer-reviewed literature, we highlight this as a caution to study teams based on the practical experience of eCOA consortium members.
In summary, the best practices for implementation start with defining a clinical concept of interest, selecting relevant digital measures, making a decision to collect point-in-time or continuous passive data, defining a number of sensors, and their respective body location with subject comfort and burden of data collection in mind, duration and frequency of data collection as well as taking steps to prevent missing data which include acceptable usability, providing training to all participants, and proactive monitoring for data compliance. We strongly recommend specific and mandatory consent be obtained for DHT-based data collection.
PARTICIPANT DATA SHARING
Historically, sharing clinical data with trial participants was not built into study design or execution, but with the advent of patient-focused drug development, the role patients play in study design, enrollment, and execution has changed. Many patients express a desire to see their own data beyond the limits of standard of care, and while data sharing with participants could be a powerful engagement tool, it can present a significant challenge. The classification of DHT-derived data as a biomarker or COA23, 30 also has implications for data sharing. In the case of COAs, FDA guidance on patient-reported outcome (PRO) measures clearly states that PROs should be collected prior to sharing any clinical data to avoid introducing biases into participant responses.81 This principle also applies to other COA categories that capture labile performance characteristics, which depend on participant motivation.11 The FDA’s guidance on DHTs for remote data acquisition in clinical trials notes that “if participants are able to view data or results from the DHT, it may impact their behavior or evaluation of the investigational product”.1 In the case of digitally measured biomarkers, data sharing does not present the same challenges because biomarkers represent normal or pathological physiology, so are not typically dependent on participant motivation, with a few exceptions, such as mobile spirometry, which does depend on participant effort to produce good quality data.48
Data sharing and its impact on data integrity in clinical trials should be considered at the stage of a study design which also includes a choice of DHTs. Some technologies do not provide end users with measurement results. However, certain technologies marketed directly to consumers, including some medical devices, such as blood pressure monitors and pulse oximeters, as well as commercial fitness trackers, may provide measurement values directly to patients. The use of DHTs with data visualization capabilities should be considered very carefully as they may introduce biofeedback and biases that could impede the evaluation of a therapeutic intervention. Ultimately, data can be shared after study completion. As evidenced by the Participant Data Return initiative launched by Pfizer, sharing individual participant data is an important tool for engaging and acknowledging patients for their contributions. Under this initiative, US trial participants will be able to register and choose to receive their data about 12 months after completion of the trial.82
To summarize, while participant data sharing can be highly desirable, the consequences need to be considered carefully. Current best practices foresee data sharing at the end of this study to avoid an impact on participant’s behavior and introducing biases.
PATIENT, CLINICIAN, AND TECHNOLOGY PROVIDER INPUT
Before finalizing the choice of DHTs, it is imperative to consider the number of sensors, body placement, and whether point-in-time and/or continuous measurement are required. Roundtable conversations bringing together key stakeholders can help substantially in making the right choices to ensure successful DHT deployment in clinical trials. Such conversations performed in public forums have demonstrated that stakeholders don’t necessarily share the same points of view, but their input is essential for value co-creation.17 In addition to defining disease features meaningful to patients, people living with the disease and/or patient advocacy organizations can provide useful insights into patients’ willingness to use a technology, potential barriers to adoption, and demonstrate the level of awareness of technology use that may be beneficial in a long term. This is especially true for pediatric populations where the caregiver input can be critical for successful DHT deployment.78 Additionally, clinician (physicians, nurses, therapists) input provides an additional perspective and enables a path forward toward the deployment in the standard of care should an investigational drug receive regulatory approval. It is important to separate these assessments from feasibility studies as proposed by Walton et al.80 These can be conducted as observational or interventional studies and are aimed at generating empirical evidence of the sites’ experience with DHTs, capturing participant experience with the DHT including usage compliance, and early assessment of data quality in terms of missing or erroneous data.
Concept elicitation studies, aimed at establishing disease features meaningful to patients, provide valuable information about disease features most important and bothersome to patients.29 At the same time, these studies may result in an opinion from a limited number of patients with diverse inputs, leading to a further challenge of how to reconcile multiple data points in the context of disease heterogeneity, especially in early disease stages when patients may present with a different set of symptoms.41 Clinicians see many patients, which empowers them to draw meaningful conclusions about the most pertinent disease features across the population. Clinicians can also provide useful insights into how DHT deployment fits in with the overall care management plan and highlight potential challenges with deployment.
Technology providers can bring not only technical expertise but also practical experience of deploying DHTs. Sensor-based DHTs come with unique requirements for deployment, due to their differences from traditional assessments in the data sources, data collection methods, frequency of assessments, specific requirements imposed by processing algorithms, definition of missing data,50 and statistical methodology.32 For example, passive continuous assessments using a sensor-based DHT may require the collection and aggregation of data from multiple days prior to dosing to derive a baseline assessment. Multiple day assessments may be required for certain concepts, such as daily step count or sleep, which require data from multiple days to establish a robust baseline. These open roundtable conversations involving sponsors, patients and patient organizations, clinicians, and technology providers are more an exception than the norm. Often conversations between sponsors and key stakeholders are held separately because of confidentiality concerns. However, most successful publicly available examples demonstrate that plurality of opinions and contributions brings the best results.16, 17
Briefly, multi-stakeholder consultations which include clinicians’ and technology providers’ input, in addition to patients’ feedback, provide a range of opinions and increase the chances of successful DHT deployment and compliant data collection.
DIVERSITY, EQUITY, AND INCLUSION
The topics of diversity, equity, and inclusion are often mentioned in the context of DHT deployment in clinical research. Given the previously mentioned potential benefits afforded by DHTs, they could aid in diversifying populations in clinical trials, consequently making study results more generalizable and providing a much-needed increase in access to trials. While the publications in peer-reviewed literature remain largely conceptual,22, 83 we provide here some considerations based on the experience of eCOA Consortium members.
An experimental design should be fit-for-purpose and take into consideration demographics of potential participants. Some experiments aimed at assessing multiple digital features and ascertaining their utility may require deployment of multiple sensors and questionnaires, combining point-in-time and continuous data collection. These experiments may be better performed at a small number of sites enrolling motivated participants who might have a higher degree of technology literacy. These initial experiments are often considered pilots. In addition to selecting variables of interest, they can also provide valuable learning experience about future deployment of devices and measures.
Experiments aimed at collecting data in ethnically and socio-demographically diverse populations can be achieved with simple solutions that require minimum effort from end users, incorporating features such as long battery life, convenient form factor, and seamless data synchronization, all often found in commercial technologies. Studies done at Montefiore Medical Center in Bronx, New York provide an example of streamlined data collection in an ethnically diverse population.84, 85 Cancer patients receiving radio- or chemoradiotherapy had an option to wear a lightweight fitness tracker before starting the therapy, throughout the treatment, and during a follow-up. The fitness tracker was waterproof, had a long battery life, and the data were synchronized automatically every time the patients attended a follow-up visit. This simple, streamlined data collection protocol resulted in high compliance of 94%. Daily step count derived from this fitness tracker was shown to be a strong dynamic predictor of hospitalizations. Additionally, the baseline activity as determined by daily step count in patients with locally advanced non-small cell lung cancer was a stronger predictor of survival than a traditionally deployed tool, the Performance Status physician rating scale.86
Additional considerations must be given when moving to scale the deployment of DHT-based assessments to a diverse population. These considerations range from analytical validation to capture technical performance in racially diverse participants to validating gait and balance algorithms for people using walking aids to adjusting to variability in body sizes. During the COVID-19 pandemic, the issues of racial discrepancies came to light when oxygen saturation levels were concurrently measured by pulse oximetry and arterial blood gas, demonstrating that pulse oximetry overestimated oxygen saturation among Asian, Black, and Hispanic patients compared with White patients as reported by Fawzy et al.87 While this publication does not report specific pulse oximetry models deployed in the study sites, a choice of a specific device model is critically important. FDA guidance on premarket notifications for pulse oximeters88 recommends testing devices in participants with different skin tones to avoid the issues described above. An independent validation study, investigating accuracy of pulse oximeters, demonstrated substantial variability among models in accuracy as related to skin tones and also data missingness.89 The other examples include variations in gait and balance for patients who are using walking aids90 and the requirements of different arm circumferences for blood pressure monitors.91
In summary, we recommend carefully considering the populations to be enrolled in the studies that include DHT-based data collection and select technologies that are fit for purpose for the specific data collection strategy required by the protocol, taking into account population demographics and potential technology literacy. There could be great variability in DHTs, and simple technological solutions best fitted for geographically and ethnically diverse populations.
SITE BURDEN AND TRAINING CONSIDERATIONS
At present, DHT-derived measures rarely replace more traditional tools, like clinician rating scales or laboratory tests; instead, they are usually implemented in addition to the traditional measures, leading to increased study complexity. This is likely to remain the status quo until enough data are collected and analyzed to allow sponsors to streamline to a DHT-centric strategy with confidence.
However, it is unlikely that sensor-based outcomes would replace the patient voice, as patient perceptions of changes in their health status in relation to a new treatment is a vital element of its long-term utility. Consequently, DHT implementation should be considered carefully with an appropriate implementation plan—if DHTs are poorly implemented, this can result in reluctance from sites to use them in trials. Protocol instructions and traditional best practices for conducting clinical studies typically suggest directing participants to contact their site for help with protocol-related questions/issues, yet the role sites play in supporting participants with respect to DHTs is yet to be adequately defined. One study suggested there are eight key characteristics contributing to site burden: comprehension, time, communication, emotional load, cognitive load, research engagement, logistical burden, and product accountability.92 These issues are augmented by the fact that there is only limited reimbursement for DHT implementation in care delivery settings,93 hampering overall adoption of DHTs. In this situation, DHT deployment in clinical trials remains the mainstream of DHT adoption, and a close collaboration among sites, sponsors, and technology providers is critical. The quality of site training has a direct impact on patient compliance as sites are the main point of contact for participants concerning technology deployment, troubleshooting, and providing feedback. Sites often report feeling they have received inadequate training and a subsequent lack of understanding of the DHT and have no immediate access to self-help tools to find solutions to participants’ questions quickly and easily. It is also key to ensure that the sites and participants understand the goal of the device inclusion, and this is central to the study design. As the number of different and varied clinical tools and DHTs expands, so does the demand from sites for training and participant support, exacerbating the site burden. The enhancement in data quality invariably comes with an increased burden on the participants and sites. This tradeoff between data and burden needs to be carefully balanced, especially for large-scale, multi-site trials, which face very different operational challenges from small or single site studies. Best practices include developing clear, step-by-step materials to train the sites, in addition to offering training sessions that provide hands-on experience with devices and related software along with refresher trainings. The phase and size of the trial may add additional requirements and are discussed below.
Early-phase studies
Early-phase studies, including Phase 0, are ideally positioned to conduct initial pilot and validation experiments with complex devices and/or deployment schedules, which may ultimately lead to a smoother implementation process and enhanced participant compliance in later phase trials. Sites may require in-person training to be comfortable with coaching participants in using DHTs. Moreover, sites must have time zone-appropriate access to DHT helpdesk support in case trial participants experience difficulties with technologies. These practices can be supplemented with concise and relevant self-help training materials, which must be reviewed on a regular basis and updated to ensure site feedback is incorporated.
Late-phase/global studies
In the context of multi-site global studies, training considerations should include consistency of materials, including translatability and scalability of training. Sometimes, a basic proof of concept study focusing on both site and participant concerns is needed to establish a global scalable model of deployment. It is important to consider that when multiple providers are involved to capture remote data, a centralized role coordinating training and communication is needed. The instructions provided by the DHT manufacturer/developer may be sufficient in certain cases for training, but they must be evaluated carefully, adjusted to the trial needs and performance of training documented. Defining participant and site support models will depend on the size and geography covered by a study; a single point of contact for each user group and escalation channels is recommended. Support models may include a combination of training modules for review and ‘how to’ documents that remain readily available to the site.
Briefly, the best practices for site training are aimed at minimizing the site burden, and include providing training materials (online or in-person training as appropriate to the study stage) to familiarize the site staff with technologies to be deployed, incorporating the feedback from the sites, and to provide helpdesk support.
COMPLIANCE MODELING
The use of computational models informing drug development has grown substantially since initial experiments in the early 1990s.94 One of the best-known approaches is model-informed drug development proposed by the FDA.95 A similar approach could be applied to sensor-based DHTs: Disease condition and DHT-derived data can be modeled to inform future study design, including anticipated compliance and data missingness. The proliferation of DHT applications in recent years has resulted in the creation of large datasets with digital components. These include natural history studies in particular indications, such as the mPower study launched by Sage Bionetworks to digitally assess disease severity in PD7 and the digital cohort of the Parkinson’s Progression Markers Initiative (PPMI),96 or observational datasets across different conditions with longitudinal follow-up, for example, the UK Biobank study.97 These datasets are publicly available and serve the important purposes of demonstrating the feasibility of digital data collection,98 characterizing standardized clinical assessments against which digital measures are usually benchmarked,99 addressing pressing medical questions such as predicting mortality risk using objective data,97 discern difficult concepts of interest such as frailty,100 or detect gait in a “walk-like activity” in PD under real-life conditions.96 In addition to these indisputable achievements, research conducted with these datasets can be very informative about patient compliance over time7 and provide good examples of computing compliance using publicly available datasets with detailed description of data processing,101 creating opportunities for data modeling to inform future study design which includes study powering and understanding data behavior to achieve predictable results.
Publicly shared datasets from natural history studies can be leveraged to model participant compliance, data missingness, and study power calculations to inform future study designs. Additionally, these datasets can be used to understand data performance and its limitations as demonstrated by the Mobilise-D project.102
CONCLUSIONS
DHT deployment practices in clinical trials continue to evolve and are sometimes still perceived as a complicating aspect of study conduct due to uncertainty with predicting participant and site burden and, consequently, participant compliance in generating the data. A somewhat slower rate of sensor-based DHT adoption has been observed than originally anticipated, despite the numerous benefits that DHT deployment can potentially offer. A variety of reasons have been suggested for this slower rate of adoption, and the lack of well-articulated best practices of ensuring participant compliance is among them. To address this gap, the member firms of the eCOA consortium reviewed literature-based evidence and augmented this evidence with their experience in supporting DHT deployment in clinical trials.
Limitations
This review has several limitations. First, the selection of topics is based on those frequently raised by eCOA Consortium members, and this selection may be biased toward members’ experience. Second, some of the proposed best practices are also based on the collective experience of the consortium members rather than systematic or scoping literature review which potentially may also introduce biases. This review did not follow the PRISMA guidelines103 which would have forced the omission of Consortium member experience and topics not covered in the peer-reviewed literature. We believe that sharing these practices based on work-related experience augments scientific knowledge and can stimulate the conception of evidence generation studies which are critical for the development of regulatory science. Third, the selection of categories is based on both the consortium member experience and the literature which may evolve over time as more data and results are published.
The proposed best practices can be separated into five main categories (Figure 1):
- Sensor and DHT measure selection. Considerations for deploying sensor-based DHT to ensure optimal participant compliance include defining a clinical concept of interest; determining whether this measure can be captured by a sensor-based DHT and digital measure selection; defining a data capture mode: point-in-time active or continuous passive data collection; deciding on a number of sensors and corresponding body location; duration of data collection and frequency of use which altogether contributes to perceived participant burden defined as a balance between participant perception of their health condition and the utility of proposed technology and its accuracy; prevention of missing data; and mandatory versus optional consent for DHT data collection.
- Participant data sharing. Sharing the DHT enabled results with participants could be another opportunity for participant engagement; however, careful consideration should be given to the data sharing’s potential impact on the clinical trial data integrity.
- Diversity, equity, and inclusion is one of the benefits of DHT adoption, though specific research aims may require input from demographically different populations. Engaging the sites by assessing DHT deployment-related site burden and developing site and participant facing materials carefully optimized for early and late-stage studies is an integral part of best practices which also include regular site feedback and feedback-based training material updates.
- Patient, clinician, and technology provider input. Establishing an open dialogue between patients and patient organizations, clinicians, and DHT technology providers ensures that the challenge of deployment can be accessed from multiple points of view and lead to value co-creation.
- Compliance modeling. Following the example of model informed drug development proposed by the FDA, uncertainty of future participant compliance can be modeled using publicly available datasets which often come from natural history studies. Several precompetitive efforts have produced empirical data from observational studies that can be used to extrapolate the results of future clinical trials and inform study power calculations.
DHT adoption rate will continue to increase as more examples of DHT-enabled study results appear in the public domain. Our proposed best practices aimed at optimizing participant compliance need to be tested holistically as an integrated approach and adjusted based on empirical evidence.
AUTHOR CONTRIBUTIONS
All authors (ESI, DM, RY, JL, KS, JK, AR, CCG, BB, and SK) have given final approval of the article and agree to be accountable for all aspects of the work in ensuring that questions related to the accuracy or integrity of any part of the work are appropriately investigated and resolved.
ACKNOWLEDGMENTS
The authors wish to thank Critical Path Institute for help with developing the manuscript and Mark Matson for critical review of the manuscript.
FUNDING INFORMATION
Support for the eCOA Consortium comes solely from membership fees paid by the members of the eCOA Consortium (https://c-path.org/program/electronic-clinical-outcome-assessment-consortium/).
CONFLICT OF INTEREST STATEMENT
ESI is an employee of Koneksa Health and may own company stock; CCG is an employee of ActiGraph LLC and may own company stock; KS is an employee of Clario and may own company stock; RY owns stocks at Medable; all other authors have nothing to declare.
- FDA: Digital Health Technologies for Remote Data Acquisition in Clinical Investigations Draft Guidance for Industry, Investigators, and Other Stakeholders. https://www.fda.gov/regulatory-information/search-fda-guidance-documents/digital-health-technologies-remote-data-acquisition-clinical-investigations. Accessed January 16, 2024.Google Scholar
- Bakker JP, Goldsack JC, Clarke M, et al. A systematic review of feasibility studies promoting the use of mobile technologies in clinical research. NPJ Digit Med. 2019; 2: 47. doi:10.1038/s41746-019-0125-xPubMedWeb of Science®Google Scholar
- Izmailova ES, Wagner JA, Perakslis ED. Wearable devices in clinical trials: hype and hypothesis. Clin Pharmacol Ther. 2018; 104: 42-52. doi:10.1002/cpt.966PubMedWeb of Science®Google Scholar
- Goldsack JC, Coravos A, Bakker JP, et al. Verification, analytical validation, and clinical validation (V3): the foundation of determining fit-for-purpose for biometric monitoring technologies (BioMeTs). NPJ Digit Med. 2020; 3: 55. doi:10.1038/s41746-020-0260-4PubMedWeb of Science®Google Scholar
- Taylor KI, Staunton H, Lipsmeier F, Nobbs D, Lindemann M. Outcome measures based on digital health technology sensor data: data- and patient-centric approaches. NPJ Digit Med. 2020; 3: 97. doi:10.1038/s41746-020-0305-8PubMedWeb of Science®Google Scholar
- Adams JL, Kangarloo T, Tracey B, et al. Using a smartwatch and smartphone to assess early Parkinson’s disease in the WATCH-PD study. NPJ Parkinsons Dis. 2023; 9: 64. doi:10.1038/s41531-023-00497-xPubMedGoogle Scholar
- Bot BM, Suver C, Neto EC, et al. The mPower study, Parkinson disease mobile data collected using ResearchKit. Sci Data. 2016; 3:160011. doi:10.1038/sdata.2016.11CASPubMedWeb of Science®Google Scholar
- Lipsmeier F, Taylor KI, Kilchenmann T, et al. Evaluation of smartphone-based testing to generate exploratory outcome measures in a phase 1 Parkinson’s disease clinical trial. Mov Disord. 2018; 33: 1287-1297. doi:10.1002/mds.27376PubMedWeb of Science®Google Scholar
- Thanarajasingam G, Kluetz PG, Bhatnagar V, et al. Integrating 4 Measures to Evaluate Physical Function in Patients with Cancer (In4M): Protocol for a prospective study. medRxiv. 2023. doi:10.1101/2023.03.08.23286924Google Scholar
- King CS, Flaherty KR, Glassberg MK, et al. A Phase-2 exploratory randomized controlled trial of INOpulse in patients with fibrotic interstitial lung disease requiring oxygen. Ann Am Thorac Soc. 2022; 19: 594-602. doi:10.1513/AnnalsATS.202107-864OCPubMedWeb of Science®Google Scholar
- Servais L, Yen K, Guridi M, Lukawy J, Vissière D, Strijbos P. Stride velocity 95th centile: insights into gaining regulatory qualification of the first wearable-derived digital endpoint for use in Duchenne muscular dystrophy trials. J Neuromuscul Dis. 2022; 9: 335-346. doi:10.3233/jnd-210743PubMedGoogle Scholar
- Bloem BR, Post E, Hall DA. An apple a day to keep the Parkinson’s disease doctor away? Ann Neurol. 2023; 93: 681-685. doi:10.1002/ana.26612PubMedWeb of Science®Google Scholar
- Garcia-Aymerich J, Puhan MA, Corriol-Rohou S, et al. Validity and responsiveness of the daily- and clinical visit-PROactive physical activity in COPD (D-PPAC and C-PPAC) instruments. Thorax. 2021; 76: 228-238. doi:10.1136/thoraxjnl-2020-214554PubMedWeb of Science®Google Scholar
- A Gene Transfer Therapy Study to Evaluate the Safety and Efficacy of Delandistrogene Moxeparvovec (SRP-9001) in Participants With Duchenne Muscular Dystrophy (DMD) (EMBARK). https://clinicaltrials.gov/study/NCT05096221. Accessed July 31, 2023.Google Scholar
- Bellerophon Announces Top-Line Data from Phase 3 REBUILD Clinical Trial of INOpulse® for Treatment of Fibrotic Interstitial Lung Disease. https://investors.bellerophon.com/news-releases/news-release-details/bellerophon-announces-top-line-data-phase-3-rebuild-clinical#:~:text=The%20primary%20endpoint%20was%20the,day%20(p%3D0.2646). Accessed July 1, 2023.Google Scholar
- Izmailova ES, Wagner JA, Ammour N, et al. Remote digital monitoring for medical product development. Clin Transl Sci. 2021; 14: 94-101. doi:10.1111/cts.12851Web of Science®Google Scholar
- Clay I, Peerenboom N, Connors DE, et al. Reverse engineering of digital measures: inviting patients to the conversation. Digit Biomark. 2023; 7: 28-44. doi:10.1159/000530413PubMedGoogle Scholar
- Izmailova ES, AbuAsal B, Hassan HE, Saha A, Stephenson D. Digital technologies: innovations that transform the face of drug development. Clin Transl Sci. 2023; 16: 1323-1330. doi:10.1111/cts.13533PubMedGoogle Scholar
- DiMasi JA, Dirks A, Smith Z, et al. Assessing the net financial benefits of employing digital endpoints in clinical trials. medRxiv 2024.2003.2007.24303937. 2024. doi:10.1101/2024.03.07.24303937Google Scholar
- EMA: Questions and answers: Qualification of digital technology-based methodologies to support approval of medicinal products. https://www.ema.europa.eu/en/documents/other/questions-answers-qualification-digital-technology-based-methodologies-support-approval-medicinal_en.pdf. Accessed July 31, 2023.Google Scholar
- Moore TJ, Heyward J, Anderson G, Alexander GC. Variation in the estimated costs of pivotal clinical benefit trials supporting the US approval of new therapeutic agents, 2015–2017: a cross-sectional study. BMJ Open. 2020; 10:e038863. doi:10.1136/bmjopen-2020-038863PubMedWeb of Science®Google Scholar
- Broadwin C, Azizi Z, Rodriguez F. Clinical trial technologies for improving equity and inclusion in cardiovascular clinical research. Cardiol Ther. 2023; 12: 215-225. doi:10.1007/s40119-023-00311-yPubMedGoogle Scholar
- Izmailova ES, Demanuele C, McCarthy M. Digital health technology derived measures: biomarkers or clinical outcome assessments? Clin Transl Sci. 2023; 16: 1113-1120. doi:10.1111/cts.13529PubMedWeb of Science®Google Scholar
- https://www.appliedclinicaltrialsonline.com/view/conflicting-terminology-in-digital-health-space-a-call-for-consensus. Accessed July 31, 2023.Google Scholar
- Olaye IM, Belovsky MP, Bataille L, et al. Recommendations for defining and reporting adherence measured by biometric monitoring technologies: systematic review. J Med Internet Res. 2022; 24:e33537. doi:10.2196/33537PubMedWeb of Science®Google Scholar
- NH: National Cancer Institute. https://www.cancer.gov/publications/dictionaries/cancer-terms/def/compliance. Accessed July 31, 2023.Google Scholar
- Ocagli H, Lorenzoni G, Lanera C, et al. Monitoring patients reported outcomes after valve replacement using wearable devices: insights on feasibility and capability study: feasibility results. Int J Environ Res Public Health. 2021; 18:7171.PubMedGoogle Scholar
- Palmcrantz S, Pennati GV, Bergling H, Borg J. Feasibility and potential effects of using the electro-dress Mollii on spasticity and functioning in chronic stroke. J Neuroeng Rehabil. 2020; 17: 109. doi:10.1186/s12984-020-00740-zPubMedGoogle Scholar
- Mammen JR, Speck RM, Stebbins GM, et al. Mapping relevance of digital measures to meaningful symptoms and impacts in early Parkinson’s disease. J Parkinsons Dis. 2023; 13: 589-607. doi:10.3233/jpd-225122PubMedWeb of Science®Google Scholar
- Vasudevan S, Saha A, Tarver ME, Patel B. Digital biomarkers: convergence of digital health technologies and biomarkers. NPJ Digit Med. 2022; 5: 36. doi:10.1038/s41746-022-00583-zPubMedWeb of Science®Google Scholar
- FDA: Biomarker Qualification: Evidentiary Framework; draft guidance for industry and FDA staff. https://www.fda.gov/regulatory-information/search-fda-guidance-documents/biomarker-qualification-evidentiary-framework. Accessed July 31, 2023.Google Scholar
- Izmailova ES, Wood WA, Liu Q, et al. Remote cardiac safety monitoring through the lens of the FDA biomarker qualification evidentiary criteria framework: a case study analysis. Digit Biomark. 2021; 5: 103-113. doi:10.1159/000515110PubMedGoogle Scholar
- Huang C, Izmailova ES, Jackson N, et al. Remote FEV1 monitoring in asthma patients: a pilot study. Clin Transl Sci. 2021; 14: 529-535. doi:10.1111/cts.12901CASPubMedWeb of Science®Google Scholar
- Kirtane KS, Jim HS, Gonzalez BD, Oswald LB. Promise of patient-reported outcomes, biometric data, and remote monitoring for adoptive cellular therapy. JCO Clin Cancer Inform. 2022; 6:e2200013. doi:10.1200/cci.22.00013PubMedGoogle Scholar
- FDA Patient-Focused Drug Development Guidance Series for Enhancing the Incorporation of the Patient’s Voice in Medical Product Development and Regulatory Decision Making. https://www.fda.gov/drugs/development-approval-process-drugs/fda-patient-focused-drug-development-guidance-series-enhancing-incorporation-patients-voice-medical. Accessed July 31, 2023.Google Scholar
- Block VJ, Bove R. We should monitor our patients with wearable technology instead of neurological examination – yes. Mult Scler. 2020; 26: 1024-1026. doi:10.1177/1352458520922762PubMedGoogle Scholar
- Gater A, Nelsen L, Coon CD, et al. Asthma Daytime Symptom Diary (ADSD) and Asthma Nighttime Symptom Diary (ANSD): measurement properties of novel patient-reported symptom measures. J Allergy Clin Immunol Pract. 2022; 10: 1249-1259. doi:10.1016/j.jaip.2021.11.026PubMedWeb of Science®Google Scholar
- Bakker JP, Izmailova ES, Clement A, et al. Regulatory pathways for qualification and acceptance of digital health technology-derived clinical trial endpoints: considerations for sponsors. Clin Pharmacol Ther. 2024. doi:10.1002/cpt.3398Google Scholar
- FitzGerald JJ, Lu Z, Jareonsettasin P, Antoniades CA. Quantifying motor impairment in movement disorders. Front Neurosci. 2018; 12: 202. doi:10.3389/fnins.2018.00202PubMedGoogle Scholar
- Ellis R, Kelly P, Huang C, Pearlmutter A, Izmailova ES. Sensor verification and analytical validation of algorithms to measure gait and balance and pronation/supination in healthy volunteers. Sensors (Basel). 2022; 22:6275 doi:10.3390/s22166275PubMedGoogle Scholar
- Mammen JR, Speck RM, Stebbins GT, et al. Relative meaningfulness and impacts of symptoms in people with early-stage Parkinson’s disease. J Parkinsons Dis. 2023; 13: 619-632. doi:10.3233/jpd-225068CASPubMedWeb of Science®Google Scholar
- Montalban X, Graves J, Midaglia L, et al. A smartphone sensor-based digital outcome assessment of multiple sclerosis. Mult Scler. 2022; 28: 654-664. doi:10.1177/13524585211028561PubMedWeb of Science®Google Scholar
- Nguyen NH, Martinez I, Atreja A, et al. Digital health technologies for remote monitoring and management of inflammatory bowel disease: a systematic review. Am J Gastroenterol. 2022; 117: 78-97. doi:10.14309/ajg.0000000000001545PubMedWeb of Science®Google Scholar
- Manta C, Jain SS, Coravos A, Mendelsohn D, Izmailova ES. An evaluation of biometric monitoring technologies for vital signs in the era of COVID-19. Clin Transl Sci. 2020; 13: 1034-1044. doi:10.1111/cts.12874CASPubMedWeb of Science®Google Scholar
- Manta C, Patrick-Lake B, Goldsack JC. Digital measures that matter to patients: a framework to guide the selection and development of digital measures of health. Digit Biomark. 2020; 4: 69-77. doi:10.1159/000509725PubMedGoogle Scholar
- Edgar CJ, Bush E(N), Adams HR, et al. Recommendations on the selection, development, and modification of performance outcome assessments: a good practices report of an ISPOR task force. Value Health. 2023; 26: 959-967. doi:10.1016/j.jval.2023.05.003PubMedGoogle Scholar
- Maetzler W, Correia Guedes L, Emmert KN, et al. Fatigue-related changes of daily function: most promising measures for the digital age. Digit Biomark. 2024; 8: 30-39. doi:10.1159/000536568PubMedGoogle Scholar
- Maher TM, Schiffman C, Kreuter M, et al. A review of the challenges, learnings and future directions of home handheld spirometry in interstitial lung disease. Respir Res. 2022; 23: 307. doi:10.1186/s12931-022-02221-4PubMedWeb of Science®Google Scholar
- Vandendriessche B, Godfrey A, Izmailova ES. Multimodal biometric monitoring technologies drive the development of clinical assessments in the home environment. Maturitas. 2021; 151: 41-47. doi:10.1016/j.maturitas.2021.06.009PubMedWeb of Science®Google Scholar
- Di J, Demanuele C, Kettermann A, et al. Considerations to address missing data when deriving clinical trial endpoints from digital health technologies. Contemp Clin Trials. 2022; 113:106661. doi:10.1016/j.cct.2021.106661PubMedWeb of Science®Google Scholar
- Izmailova ES, Ellis RD. When work hits home: the cancer-treatment journey of a clinical scientist driving digital medicine. JCO Clin Cancer Inform. 2022; 6:e2200033. doi:10.1200/cci.22.00033PubMedWeb of Science®Google Scholar
- Hill DL, Stephenson D, Brayanov J, et al. Metadata framework to support deployment of digital health technologies in clinical trials in Parkinson’s disease. Sensors (Basel). 2022; 22:2136. doi:10.3390/s22062136CASPubMedGoogle Scholar
- Fredriksson T, Kemp Gudmundsdottir K, Frykman V, et al. Intermittent vs continuous electrocardiogram event recording for detection of atrial fibrillation-compliance and ease of use in an ambulatory elderly population. Clin Cardiol. 2020; 43: 355-362. doi:10.1002/clc.23323PubMedWeb of Science®Google Scholar
- King RC, Villeneuve E, White RJ, Sherratt RS, Holderbaum W, Harwin WS. Application of data fusion techniques and technologies for wearable health monitoring. Med Eng Phys. 2017; 42: 1-12. doi:10.1016/j.medengphy.2016.12.011PubMedWeb of Science®Google Scholar
- Izmailova ES, McLean IL, Hather G, et al. Continuous monitoring using a wearable device detects activity-induced heart rate changes after administration of amphetamine. Clin Transl Sci. 2019; 12: 677-686. doi:10.1111/cts.12673CASPubMedWeb of Science®Google Scholar
- Horak FB, Mancini M. Objective biomarkers of balance and gait for Parkinson’s disease using body-worn sensors. Mov Disord. 2013; 28: 1544-1551. doi:10.1002/mds.25684PubMedWeb of Science®Google Scholar
- Desai R, Blacutt M, Youdan G, et al. Postural control and gait measures derived from wearable inertial measurement unit devices in Huntington’s disease: recommendations for clinical outcomes. Clin Biomech (Bristol, Avon). 2022; 96:105658. doi:10.1016/j.clinbiomech.2022.105658PubMedGoogle Scholar
- Sotirakis C, Conway N, Su Z, et al. Longitudinal monitoring of progressive supranuclear palsy using body-worn movement sensors. Mov Disord. 2022; 37: 2263-2271. doi:10.1002/mds.29194PubMedWeb of Science®Google Scholar
- Curtze C, Nutt JG, Carlson-Kuhta P, Mancini M, Horak FB. Levodopa is a double-edged sword for balance and gait in people with Parkinson’s disease. Mov Disord. 2015; 30: 1361-1370. doi:10.1002/mds.26269CASPubMedWeb of Science®Google Scholar
- Prasanth H, Caban M, Keller U, et al. Wearable sensor-based real-time gait detection: a systematic review. Sensors. 2021; 21: 2727.Google Scholar
- Sotirakis C, Su Z, Brzezicki MA, et al. Identification of motor progression in Parkinson’s disease using wearable sensors and machine learning. Npj Parkinson’s Dis. 2023; 9: 142. doi:10.1038/s41531-023-00581-2PubMedGoogle Scholar
- Shah VV, Rodriguez-Labrada R, Horak FB, et al. Gait variability in spinocerebellar ataxia assessed using wearable inertial sensors. Mov Disord. 2021; 36: 2922-2931. doi:10.1002/mds.28740PubMedWeb of Science®Google Scholar
- Czech M, Demanuele C, Erb MK, et al. The impact of reducing the number of wearable devices on measuring gait in Parkinson disease: noninterventional exploratory study. JMIR Rehabil Assist Technol. 2020; 7:e17986. doi:10.2196/17986PubMedGoogle Scholar
- Byrom B, Rowe DA. Measuring free-living physical activity in COPD patients: deriving methodology standards for clinical trials through a review of research studies. Contemp Clin Trials. 2016; 47: 172-184. doi:10.1016/j.cct.2016.01.006PubMedWeb of Science®Google Scholar
- Bouten CV, Sauren AA, Verduin M, Janssen JD. Effects of placement and orientation of body-fixed accelerometers on the assessment of energy expenditure during walking. Med Biol Eng Comput. 1997; 35: 50-56. doi:10.1007/bf02510392CASPubMedWeb of Science®Google Scholar
- Micó-Amigo ME, Bonci T, Paraschiv-Ionescu A, et al. Assessing real-world gait with digital technology? Validation, insights and recommendations from the mobilise-D consortium. J Neuroeng Rehabil. 2023; 20: 78. doi:10.1186/s12984-023-01198-5PubMedGoogle Scholar
- Scott JJ, Rowlands AV, Cliff DP, Morgan PJ, Plotnikoff RC, Lubans DR. Comparability and feasibility of wrist- and hip-worn accelerometers in free-living adolescents. J Sci Med Sport. 2017; 20: 1101-1106. doi:10.1016/j.jsams.2017.04.017PubMedWeb of Science®Google Scholar
- Turner-McGrievy GM, Dunn CG, Wilcox S, et al. Defining adherence to mobile dietary self-monitoring and assessing tracking over time: tracking at least two eating occasions per day is best marker of adherence within two different mobile health randomized weight loss interventions. J Acad Nutr Diet. 2019; 119: 1516-1524. doi:10.1016/j.jand.2019.03.012PubMedWeb of Science®Google Scholar
- Hauguel-Moreau M, Naudin C, N’Guyen L, et al. Smart bracelet to assess physical activity after cardiac surgery: a prospective study. PLoS One. 2020; 15:e0241368. doi:10.1371/journal.pone.0241368CASPubMedGoogle Scholar
- Chung IY, Jung M, Lee SB, et al. An assessment of physical activity data collected via a smartphone app and a smart band in breast cancer survivors: observational study. J Med Internet Res. 2019; 21: 13463. doi:10.2196/13463PubMedWeb of Science®Google Scholar
- Roussos G, Herrero TR, Hill DL, et al. Identifying and characterising sources of variability in digital outcome measures in Parkinson’s disease. NPJ Digit Med. 2022; 5: 93. doi:10.1038/s41746-022-00643-4PubMedWeb of Science®Google Scholar
- McCarthy M, Bury DP, Byrom B, Geoghegan C, Wong S. Determining minimum wear time for mobile sensor technology. Ther Innov Regul Sci. 2021; 55: 33-37. doi:10.1007/s43441-020-00187-3PubMedGoogle Scholar
- Buekers J, , Marchena J, Koch S, et al. How many hours and days are needed? Digital assessment of walking activity and gait in adults with walking impairment. Mobilise-D Conference March 20, 2024.Google Scholar
- Shah V, Muzyka D, Guidarelli C, Sowalsky K, Winters-Stone KM, Horak FB. Abstract 4397: Chemotherapy-induced peripheral neuropathy and falls in cancer survivors relate to digital balance and gait impairments. Cancer Res. 2023; 83:4397. doi:10.1158/1538-7445.Am2023-4397Google Scholar
- Smith MT, McCrae CS, Cheung J, et al. Use of actigraphy for the evaluation of sleep disorders and circadian rhythm sleep-wake disorders: an American Academy of Sleep Medicine Clinical Practice Guideline. J Clin Sleep Med. 2018; 14: 1231-1237. doi:10.5664/jcsm.7230PubMedWeb of Science®Google Scholar
- Scotina AD, Lavine JS, Izmailova ES, et al. Objective physical activity measures to predict hospitalizations among patients with cancer receiving chemoradiotherapy. JCO Oncol Pract. 2023; 19:592. doi:10.1200/OP.2023.19.11_suppl.592Google Scholar
- Burq M, Rainaldi E, Ho KC, et al. Virtual exam for Parkinson’s disease enables frequent and reliable remote measurements of motor function. NPJ Digit Med. 2022; 5: 65. doi:10.1038/s41746-022-00607-8PubMedWeb of Science®Google Scholar
- Bruno E, Simblett S, Lang A, et al. Wearable technology in epilepsy: the views of patients, caregivers, and healthcare professionals. Epilepsy Behav. 2018; 85: 141-149. doi:10.1016/j.yebeh.2018.05.044PubMedWeb of Science®Google Scholar
- Whitelaw S, Pellegrini DM, Mamas MA, Cowie M, Van Spall HGC. Barriers and facilitators of the uptake of digital health technology in cardiovascular care: a systematic scoping review. Eur Heart J Digit Health. 2021; 2: 62-74. doi:10.1093/ehjdh/ztab005PubMedGoogle Scholar
- Walton MK, Cappelleri JC, Byrom B, et al. Considerations for development of an evidence dossier to support the use of mobile sensor technology for clinical outcome assessments in clinical trials. Contemp Clin Trials. 2020; 91:105962. doi:10.1016/j.cct.2020.105962CASPubMedWeb of Science®Google Scholar
- FDA: Patient-reported outcome measures: use in medical product development to support labeling claims guidance for industry. https://www.fda.gov/regulatory-information/search-fda-guidance-documents/patient-reported-outcome-measures-use-medical-product-development-support-labeling-claims. Accessed July 31, 2023.Google Scholar
- Clinical leader, September 21, 2023: Pfizer to provide individual participant data at scale. https://www.clinicalleader.com/doc/pfizer-to-provide-individual-participant-data-at-scale-0001. Accessed October 26, 2023.Google Scholar
- Fashoyin-Aje LA, Tendler C, Lavery B, et al. Driving diversity and inclusion in cancer drug development – industry and regulatory perspectives, current practices, opportunities, and challenges. Clin Cancer Res. 2023; 29: 3566-3572. doi:10.1158/1078-0432.Ccr-23-1391PubMedGoogle Scholar
- Izmailova E, Huang C, Cantor M, Ellis R, Ohri N. Daily step counts to predict hospitalizations during concurrent chemoradiotherapy for solid tumors. J Clin Oncol. 2019; 37:293. doi:10.1200/JCO.2019.37.27_suppl.293Web of Science®Google Scholar
- Ohri N, Kabarriti R, Bodner WR, et al. Continuous activity monitoring during concurrent chemoradiotherapy. Int J Radiat Oncol Biol Phys. 2017; 97: 1061-1065. doi:10.1016/j.ijrobp.2016.12.030PubMedWeb of Science®Google Scholar
- West HJ, Jin JO. JAMA oncology patient page. Performance status in patients with cancer. JAMA Oncol. 2015; 1: 998. doi:10.1001/jamaoncol.2015.3113PubMedWeb of Science®Google Scholar
- Fawzy A, Wu TD, Wang K, et al. Racial and ethnic discrepancy in pulse oximetry and delayed identification of treatment eligibility among patients with COVID-19. JAMA Intern Med. 2022; 182: 730-738. doi:10.1001/jamainternmed.2022.1906CASPubMedWeb of Science®Google Scholar
- Pulse Oximeters – Premarket Notification Submissions [510(k)s]: Guidance for Industry and Food and Drug Administration Staff. https://www.fda.gov/regulatory-information/search-fda-guidance-documents/pulse-oximeters-premarket-notification-submissions-510ks-guidance-industry-and-food-and-drug. Accessed October 26, 2023.Google Scholar
- Jiang Y, Spies C, Magin J, Bhosai SJ, Snyder L, Dunn J. Investigating the accuracy of blood oxygen saturation measurements in common consumer smartwatches. PLoS Digit Health. 2023; 2:e0000296. doi:10.1371/journal.pdig.0000296PubMedGoogle Scholar
- Lang CE, Barth J, Holleran CL, Konrad JD, Bland MD. Implementation of wearable sensing technology for movement: pushing forward into the routine physical rehabilitation care field. Sensors (Basel). 2020; 20:5744. doi:10.3390/s20205744PubMedGoogle Scholar
- Muntner P, Shimbo D, Carey RM, et al. Measurement of blood pressure in humans: A scientific statement from the American Heart Association. Hypertension. 2019; 73: e35-e66. doi:10.1161/hyp.0000000000000087CASPubMedWeb of Science®Google Scholar
- Donnelly S, Reginatto B, Kearns O, et al. The burden of a remote trial in a nursing home setting: qualitative study. J Med Internet Res. 2018; 20:e220. doi:10.2196/jmir.9638PubMedWeb of Science®Google Scholar
- Izmailova ES, Wood WA. Biometric monitoring technologies in cancer: the past, present, and future. JCO Clin Cancer Inform. 2021; 5: 728-733. doi:10.1200/cci.21.00019PubMedWeb of Science®Google Scholar
- Madabushi R, Seo P, Zhao L, Tegenge M, Zhu H. Review: Role of model-informed drug development approaches in the lifecycle of drug development and regulatory decision-making. Pharm Res. 2022; 39: 1669-1680. doi:10.1007/s11095-022-03288-wCASPubMedWeb of Science®Google Scholar
- Kuemmel C, Yang Y, Zhang X, et al. Consideration of a credibility assessment framework in model-informed drug development: potential application to physiologically-based pharmacokinetic modeling and simulation. CPT Pharmacometrics Syst Pharmacol. 2020; 9: 21-28. doi:10.1002/psp4.12479CASPubMedWeb of Science®Google Scholar
- Atri R, Urban K, Marebwa B, et al. Deep learning for daily monitoring of Parkinson’s disease outside the clinic using wearable sensors. Sensors (Basel). 2022; 22:6831. doi:10.3390/s22186831PubMedGoogle Scholar
- Zhou H, Zhu R, Ung A, Schatz B. Population analysis of mortality risk: predictive models from passive monitors using motion sensors for 100,000 UK biobank participants. PLoS Digit Health. 2022; 1:e0000045. doi:10.1371/journal.pdig.0000045PubMedGoogle Scholar
- Schneider RB, Omberg L, Macklin EA, et al. Design of a virtual longitudinal observational study in Parkinson’s disease (AT-HOME PD). Ann Clin Transl Neurol. 2021; 8: 308-320. doi:10.1002/acn3.51236CASPubMedWeb of Science®Google Scholar
- Evers LJW, Krijthe JH, Meinders MJ, Bloem BR, Heskes TM. Measuring Parkinson’s disease over time: the real-world within-subject reliability of the MDS-UPDRS. Mov Disord. 2019; 34: 1480-1487. doi:10.1002/mds.27790PubMedWeb of Science®Google Scholar
- Jiang R, Noble S, Sui J, et al. Associations of physical frailty with health outcomes and brain structure in 483 033 middle-aged and older adults: a population-based study from the UK biobank. Lancet Digit Health. 2023; 5: e350-e359. doi:10.1016/s2589-7500(23)00043-2CASPubMedGoogle Scholar
- Doherty A, Jackson D, Hammerla N, et al. Large scale population assessment of physical activity using wrist worn accelerometers: the UK biobank study. PLoS One. 2017; 12:e0169649. doi:10.1371/journal.pone.0169649PubMedWeb of Science®Google Scholar
- Access Mobilise-D Research Data. https://mobilise-d.eu/data/. Accessed July 25, 2024.Google Scholar
- Tricco AC, Lillie E, Zarin W, et al. PRISMA Extension for Scoping Reviews (PRISMA-ScR): checklist and explanation. Ann Intern Med. 2018; 169: 467-473. doi:10.7326/m18-0850PubMedGoogle Scholar
- Lipsmeier F, Taylor KI, Postuma RB, et al. Reliability and validity of the Roche PD Mobile Application for remote monitoring of early Parkinson’s disease. Sci Rep. 2022; 12: 12081. doi:10.1038/s41598-022-15874-4CASPubMedWeb of Science®Google Scholar